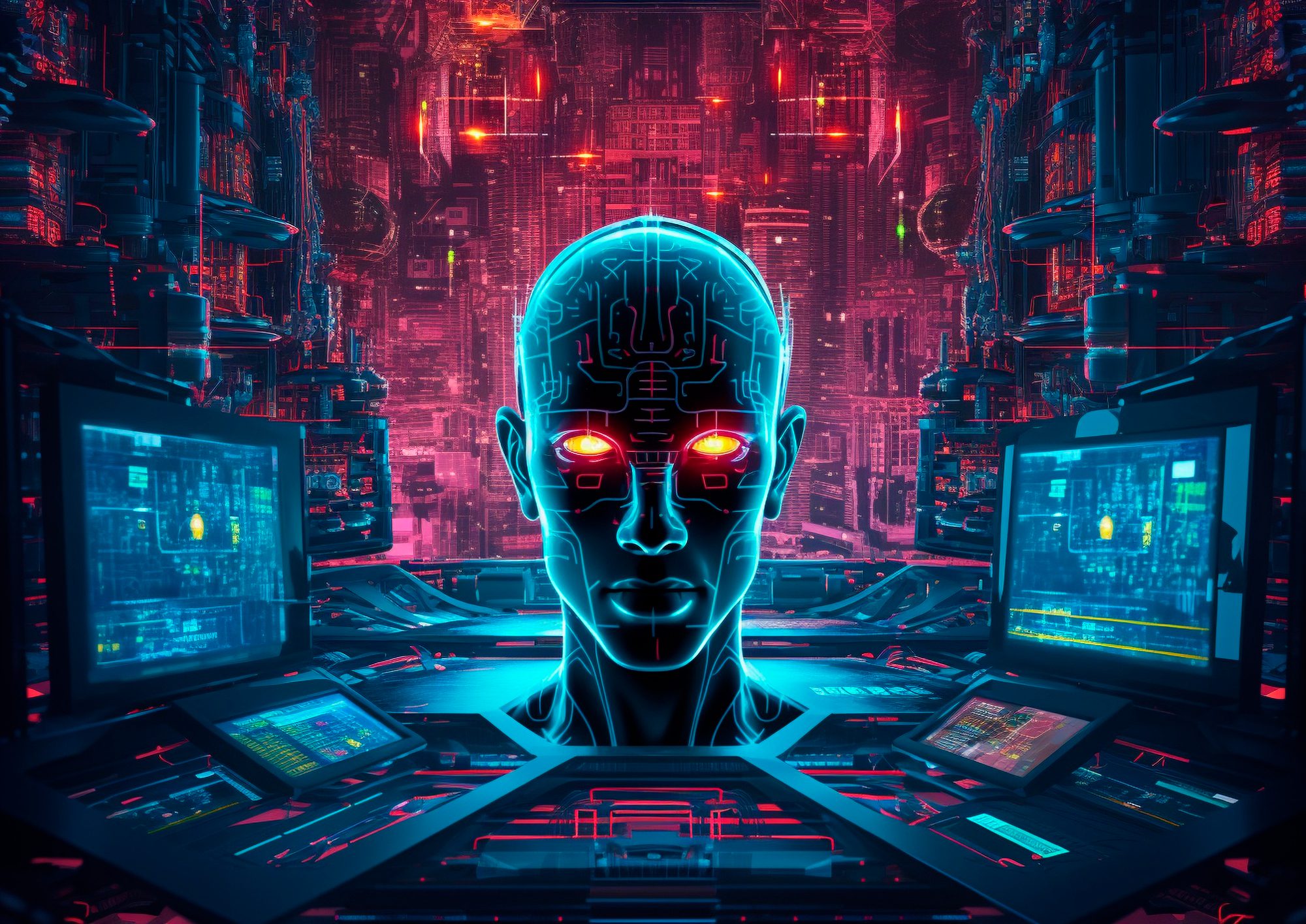
Data Science for Predictive Maintenance in Manufacturing
Enhancing Manufacturing Efficiency through Data-Driven Predictive Maintenance
Skills you will gain:
About Workshop:
This workshop focuses on using data science and AI for predictive maintenance in the manufacturing industry. Participants will learn how to build predictive models, analyze equipment data, and prevent failures through early detection. The program includes hands-on experience with real-world industrial datasets.
Aim: To equip PhD scholars and academicians with advanced skills in data science and AI techniques to predict equipment failure in manufacturing, reducing downtime and costs. The course emphasizes predictive maintenance using data-driven approaches to improve operational efficiency.
Workshop Objectives:
- Implement data science techniques to predict equipment failures.
- Build machine learning models for predictive maintenance.
- Integrate IoT data for real-time equipment monitoring.
- Analyze manufacturing data to optimize maintenance schedules.
- Gain hands-on experience with real-world datasets in predictive maintenance.
What you will learn?
Day 1: Introduction to Predictive Maintenance
Duration: 1 Hour
Objective: Provide a foundational understanding of predictive maintenance within the manufacturing sector.
Session Details:
- Overview of Predictive Maintenance: Definition, importance, and how it compares to reactive and preventative maintenance.
- Data Collection in Manufacturing: Types of data collected from manufacturing equipment that are crucial for predictive analysis.
- Introduction to Data Science Tools: Brief on tools and software that will be used throughout the course (e.g., Python, R, specific libraries for machine learning).
- Hands-On Activity: Setting up the data science environment and exploring initial datasets.
Day 2: Machine Learning for Equipment Failure Prediction
Duration: 1 Hour
Objective: Dive into machine learning techniques that enable the prediction of equipment failures.
Session Details:
- Feature Engineering: Identifying and engineering features from manufacturing data that are predictive of equipment health.
- Building Predictive Models: Introduction to key machine learning algorithms used in predictive maintenance (e.g., regression analysis, decision trees, neural networks).
- Model Training and Validation: Steps to train models and validate their accuracy using real or simulated data.
- Hands-On Activity: Participants will build a simple predictive model using a provided dataset.
Day 3: Implementing and Optimizing Predictive Maintenance Models
Duration: 1 Hour
Objective: Learn to implement, deploy, and optimize predictive maintenance models in a manufacturing setting.
Session Details:
- Model Deployment: How to deploy models into production environments safely and efficiently.
- Performance Monitoring and Optimization: Techniques for monitoring model performance over time and optimizing them for better accuracy and reliability.
- Case Studies: Discussion of successful predictive maintenance implementations in the industry.
- Hands-On Activity: Using their models, participants will simulate deploying them in a production-like environment and monitor their outputs.
Mentor Profile
Fee Plan
Important Dates
05 Nov 2024 Indian Standard Timing 1:00 pm
05 Nov 2024 to 07 Nov 2024 Indian Standard Timing 5 PM
Get an e-Certificate of Participation!
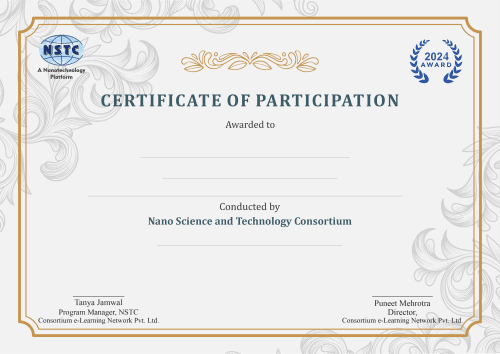
Intended For :
Data scientists, AI professionals, manufacturing engineers, and academic researchers.
Career Supporting Skills
Workshop Outcomes
- Build and implement predictive maintenance models.
- Use AI techniques to predict and prevent equipment failures.
- Analyze and process IoT and sensor data for real-time monitoring.
- Apply machine learning algorithms to optimize manufacturing operations.
- Design a comprehensive predictive maintenance system using data science.